Extracting Data from PDF Files with Python and PDFQuery
How to read and extract data from PDF files
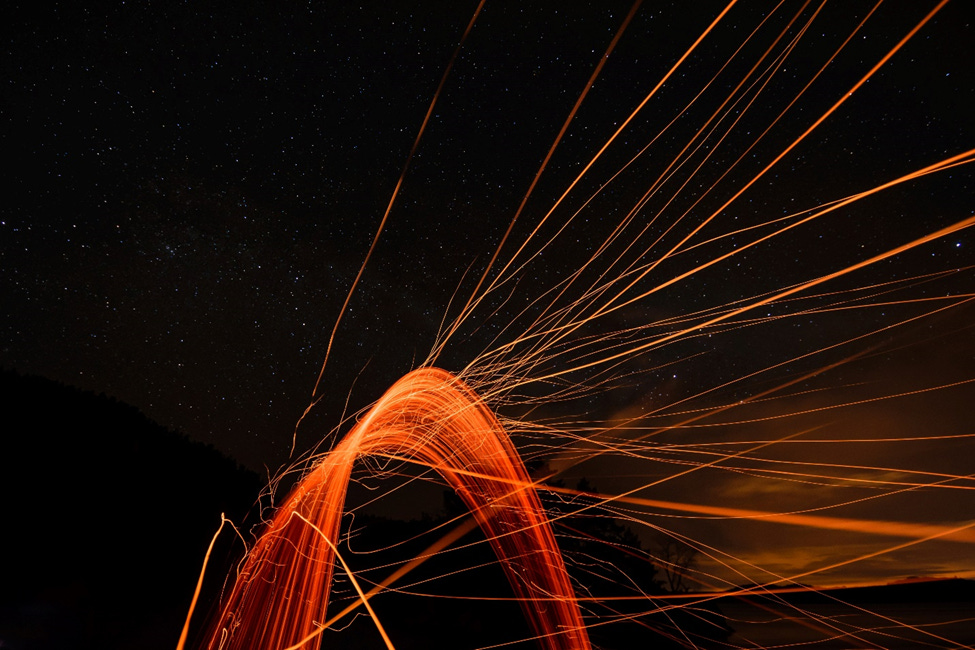
Today there is an enormous amount of data stored in PDF files and there is increasingly a need to have the data migrated from the PDF files into a structured dataframe or database for analysis and discovery. Manual data entry from PDF files to a structured dataframe would require a lot of time, resources, and expense. Alternatively, you can automate this process by using one of the Python libraries to read and extract data and store the data in a structured format.
There are several Python libraries that can be used to read and extract data from PDF files. These include PDFMiner, PyPDF2, PDFQuery and PyMuPDF. For this project we will use PDFQuery to read and extract data from multiple PDF files. PDFQuery reads a PDF file as an object, converts the PDF object to an XML file, and accesses the desired information by its specific location inside of the PDF document.
In this article we will create a script that will read PDF files, extract some data elements, and create a structured dataset. The following steps will be performed using Python and PDFQuery.
1. Install the Packages.
2. Import the Libraries.
3. Upload the PDF files.
4. Read and Convert the PDF Files.
5. Access and Extract the Data.
6. View the Dataframe.
Install the Packages
To start we will need to install pdfquery and pandas packages and import the libraries.
!pip install pdfquery
!pip install pandas
Import the Libraries
import pdfquery
import pandas as pd
PDFQuery is a light wrapper around pdfminer, lxml and pyquery. It is designed to read and extract data from multiple PDF files with as little code as possible. PDFQuery works by loading a PDF as a pdfminer layout, converting the layout to an etree with lxml.etree, and then applying a pyquery wrapper. All three underlying libraries are exposed, so you can use any of their interfaces to get at the data you want.
Pandas is an open-source library that provides high-performance data analysis and manipulation.
Upload the PDF Files
We are developing in the Google colab environment so we need to load the PDF files from the local drive to the colab drive. For this project we will upload three PDF files which can be found in Github site here.
# Upload pdf files from the local drive.
from google.colab import files
uploaded = files.upload()
Shown below is an image of one of the PDF files.
Read and Convert the PDF Files
We will now read one of the pdf files as an element object and load it.
pdf = pdfquery.PDFQuery('patient1pdf.pdf')
pdf.load()
Next we need to convert the pdf object into an Extensible Markup Language (XML) file. This file will include data and metadata for a given pdf page.
pdf.tree.write('patient1xml.xml', pretty_print = True)
pdf
XML defines a set of rules for encoding PDF in a format that is readable by humans and machines. We need to look at the XML file with a text editor to get the information we need for the data we want to extract. I prefer using Sublime Text editor to look at the XML file, however you can use any text editor you want.
The following is a fragment of the XML file for Patient Name. It includes both data (John Jacob) and metadata (e.g., text box coordination, height, width, etc.)
<LTTextLineHorizontal y0="543.657" y1="554.657" x0="72.0" x1="205.474" width="133.474"
height="11.0" bbox="[72.0, 543.657, 205.474, 554.657]" word_margin="0.1"><LTTextBoxHorizontal
y0="543.657" y1="554.657" x0="72.0" x1="205.474" width="133.474" height="11.0" bbox=
"[72.0, 543.657, 205.474, 554.657]" index="3">Patient Name: John Jacob </LTTextBoxHorizontal></LTTextLineHorizontal>
We can see the information we are looking for inside the ‘LTTextBoxHorizontal’ tag, and we can see the metadata associated with it. One way we can access this data is by using its bounding box or ‘in_bbox’. The values inside the text box, [72.0, 543.657, 205.474, 554,657] in the XML fragment refers to “Left, Bottom, Right, Top” coordinates of the text box. You can think of this as the boundaries around the data we want to extract (patient name in this case).
Access and Extract Data
Let’s access and extract the patient name using the coordinates of the text box.
patient_name = pdf.pq('LTTextLineHorizontal:in_bbox("72.0, 543.657, 205.474, 554.657")').text()
patient_name
‘Patient Name: John Jacob’
Sometimes the data we want to extract is not in the exact same location in every file which can cause issues. Fortunately, PDFQuery can also query tags that contain a given string. Let’s get the patients name by using this method. We will first clean the text data up using the text.split method. The split() method breaks up a string at the specified separator (:) and returns a list of strings (John Jacob).
def clean_text_data(text):
return text.split(':')[1]
patient_name = clean_text_data(pdf.pq('LTTextLineHorizontal:contains("Patient Name")').text())
patient_name
‘John Jacob’
Putting it all together…
We will now use the methods described above to create a pandas dataframe from multiple PDF files. For this project we will only use 3 PDF files but you could process thousands of files if needed. We will write a script that reads some PDF files, loads the files, and extracts and captures the data elements. We will then create a new dataframe.
import pdfquery
import pandas as pd
pdf_files = ['patient1pdf.pdf','patient2pdf.pdf','patient3pdf.pdf']
def clean_text_data(text):
return text.split(':')[1]
def pdf_to_df(pdf_files):
patient_data = {"Patient Number": [],
"Patient Name": [],
"DOB": [],
"Diagnosis": [],
"Treatment": [],
"Recommendation": []}
for i in pdf_files:
pdf = pdfquery.PDFQuery(i)
pdf.load()
patient_data["Patient Number"].append(clean_text_data(pdf.pq('LTText\LineHorizontal:contains("Patient Number")').text()))
patient_data["Patient Name"].append(clean_text_data(pdf.pq('LTText\LineHorizontal:contains("Patient Name")').text()))
patient_data["DOB"].append(clean_text_data(pdf.pq('LTText\LineHorizontal:contains("DOB")').text()))
patient_data["Diagnosis"].append(clean_text_data(pdf.pq('LTText\LineHorizontal:contains("Diagnosis")').text()))
patient_data["Treatment"].append(clean_text_data(pdf.pq('LTText\LineHorizontal:contains("Treatment")').text()))
patient_data["Recommendation"].append(clean_text_data(pdf.pq('LTText\LineHorizontal:contains("Recommendation")').text()))
columns=["Patient Number","Patient Name","DOB","Diagnosis","Treatment","Recommendation"]
pdata = pd.DataFrame.from_dict(patient_data)
pdata = pdata[columns]
return pdata
pdata = pdf_to_df(pdf_files)
View the Dataframe
Let’s look at the new dataframe containing the data elements from the PDF files.
pdata
This script shows how you can easily extract data from multiple PDF files and create a new structured dataframe. This structured data set can then be used for further analysis and discovery.
Thanks so much for reading my article! If you have any comments or feedback, please add them below.